Best Practices
With Machine Learning, Predictive Modeling for Admissions Is Evolving

by JC Bonilla · Jan 22, 2021
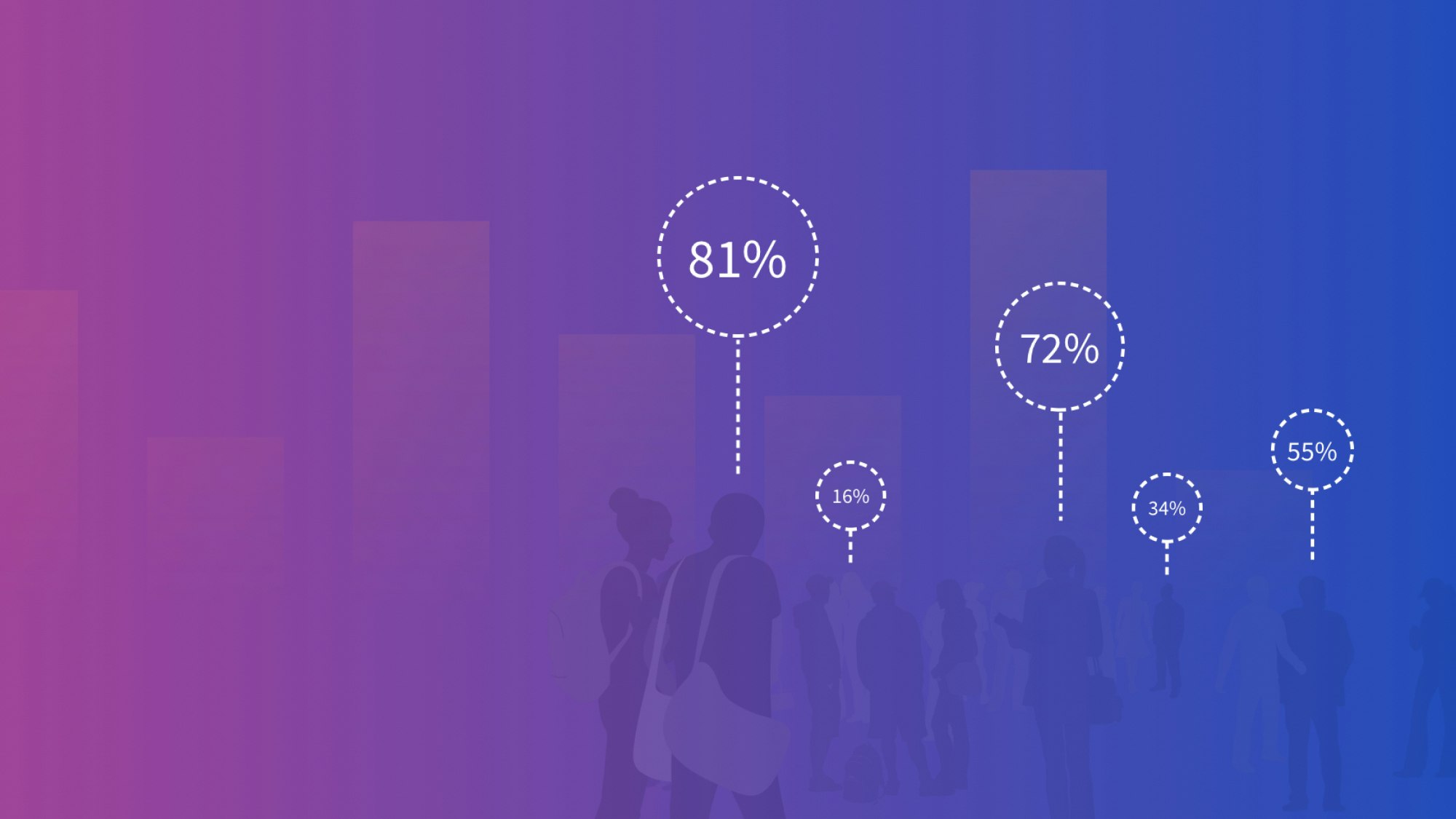
Loading...
Loading...
Loading...
Loading...
Loading...
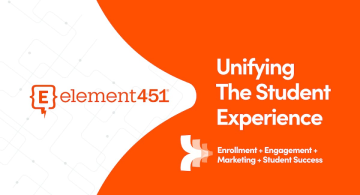
About Element451
Element451 is the leading Student Engagement platform for higher education. Go from noise to relevance with timely, personalized communication on every channel. Welcome to the era of student-centric engagement.
Categories
AI
Applications
Automation
Best Practices
Case Studies
Content
Events
Marketing
News & Updates
Personalization
Product
UX & Design
New Blog Posts
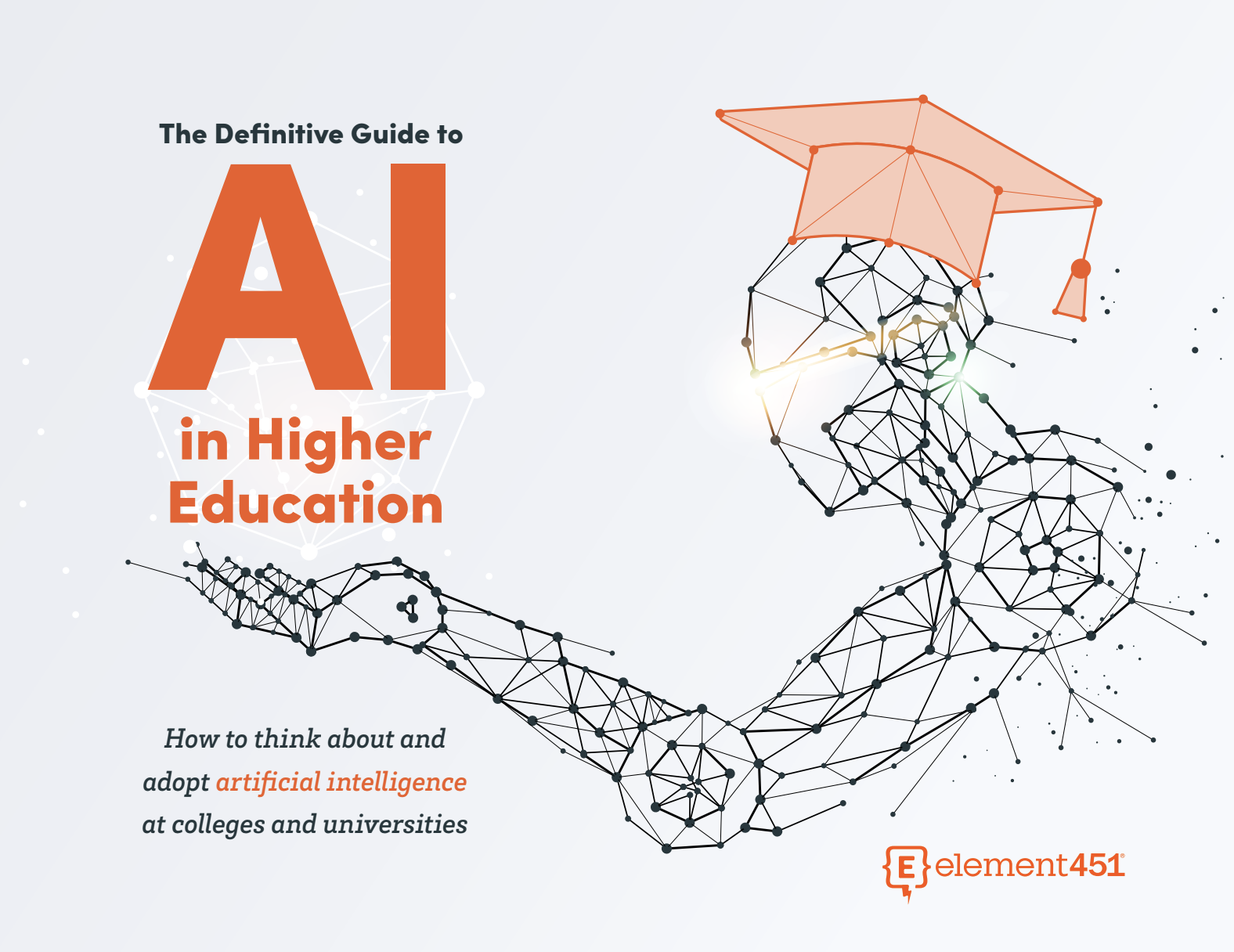
The Definitive Guide
AI in Higher Education
Bridge the gap between the latest tech advancements and your institution's success.
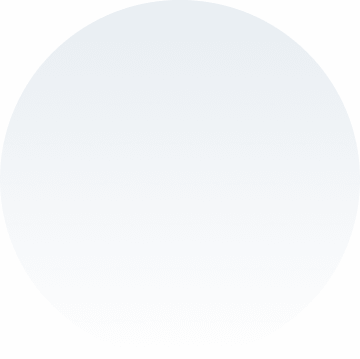
Talk With Us
Element451 is the only AI-first CRM and Student Engagement platform for higher education. Our friendly experts are here to help you explore how Element451 can improve outcomes for your school.
Get a Demo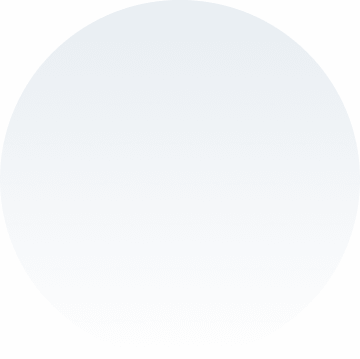
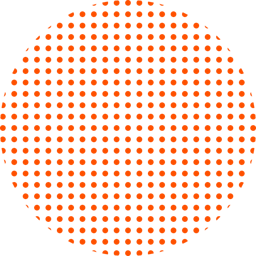
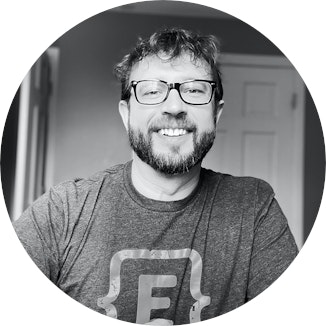